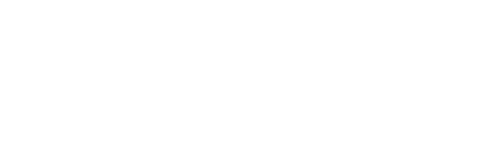
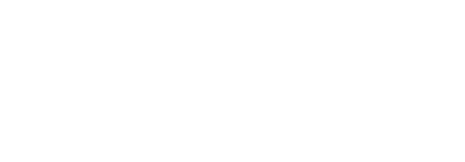
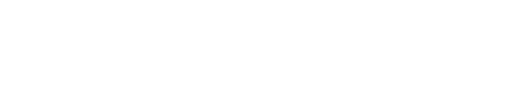
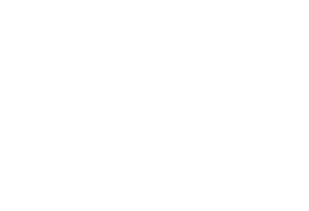
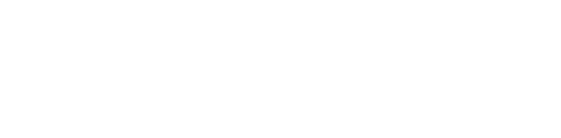
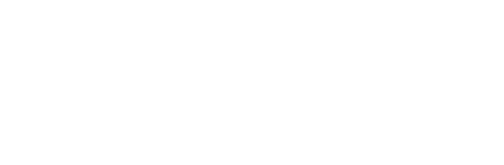
Discover the valuable insights our consultants provide. Subscribe to our Insights blog to receive email alerts whenever we post something new!
Published on: May 28, 2024 8:23:40 AM by Jacob McClendon
Updated on: March 20, 2025
Organizations are increasingly recognizing the value of high-quality data for making informed decisions, operating efficiently, enhancing customer experiences, and building a competitive edge. However, achieving and maintaining high-quality data requires robust data governance practices, which can take time to develop and deploy.
Here, we explore essential practices for data governance to ensure data quality, providing a comprehensive guide for organizations aiming to optimize their data assets.
Implementing effective data governance is foundational for organizations to harness the full potential of their data assets. It not only ensures data quality, but also supports regulatory compliance and enhances overall business efficiency.
At its core, data governance is about ensuring that data is accurate, managed consistently, secured, and available for authorized users. A set of processes, policies, and standards are established to ensure the effective and efficient use of information, helping organizations achieve data-driven decision-making and efficient operations.
Data governance is neccesary for several reasons:
Effective data governance involves several key components. Establishing proper roles and responsibilities ensures the organization is aligned on ownership and direction, and ultimately who is accountable.
Defining policies, standards, and management practices ensures the organization has a guide for data management practices. Let’s explore each.
Data owners, stewards, and custodians collaborate closely as each role brings unique skill sets to the team. Each role enhances data quality.
Data Owners are responsible for the overall management and oversight of a specific data asset. This role typically falls to a senior business executive or department head who has the authority to make decisions about data usage and policies. They help define policies and standards, access and usage permissions, and any approval or prioritization decisions.
Data Stewards oversee the daily management and oversight of data assets. They designate individuals or teams to be accountable for data management, quality, metadata, policy implementation, and issue resolution.
Data Custodians are responsible for the technical aspects of managing data assets, such as data storage, security, infrastructure, and system performance. They have deep technical expertise in data management technologies and security practices.
Developing clear and comprehensive data policies is essential for guiding data management practices. These policies should define standards for data quality, data privacy, data security, and data usage, with detailed requirements for each of the above.
Implementing processes and tools to monitor and improve data quality is a must for maintaining accurate, complete, and reliable data. Data quality management involves data profiling, data cleansing, and data enrichment, and once the standards are established, can be built into existing data infrastructure.
Many of the quality management tasks can be implemented during the design of a database or system and can be automated.
Data quality management is a core component of data governance. Let’s explore key data quality management activities.
Analyzing data to understand its structure, content (i.e., columns in a table and their ranges or value sets), and quality is the first step in data quality management. Data profiling helps identify data issues such as missing or incorrect values, duplicate records, and inconsistencies. Some cloud database tools like Snowflake have profiling tools built in when you query a table, and you can also leverage data visualization tools like Tableau or Power BI to look at your data.
Correcting data issues identified during data profiling is essential for improving data quality. Data cleansing involves standardizing data formats, removing duplicates, filling in missing values, and replacing or modifying values.
Enhancing data by adding relevant information from additional sources or calculations, models, or generative methods like AI can improve data quality and provide more valuable insights. Tools like Matillion offer quick methods to seamlessly bring in additional data and leverage AI right where your data is already.
Maintaining high standards requires continuous monitoring of data quality. Implementing data quality dashboards and automated data quality checks can help identify and address data issues promptly rather than manually determining if quality is degrading over time. As an example, we typically establish dashboards to surface data pipeline run errors with automated email alerts if an error occurs.
Insight: Breaking Down Data Silos: Why Data Integration Matters
Data integrity refers to the accuracy and consistency of data over its lifecycle. Ensuring data integrity is critical for maintaining trust in the data and making reliable business decisions. Here are some key practices for ensuring data integrity.
Ensure that data meets predefined standards and business rules. Implementing validation rules and checks within source systems and during data processing can limit errors and irregularities from entering downstream visualizations or applications.
Conducting regular data audits helps identify data integrity issues and ensures adherence to data governance policies. Your governance policies and standards should define what to audit and how frequently.
Maintaining version control of data and infrastructure solutions helps track changes and ensures that both data and infrastructure remain consistent over time. Data versioning involves creating snapshots of data, infrastructure, or entire systems at different points in time and maintaining a history of data and infrastructure changes.
Implementing and periodically testing data backup and recovery procedures ensures that data can be restored in case of data loss or corruption.
Implementing best practices for data governance is essential for ensuring data quality. Let’s look at some of the common practices.
Developing a comprehensive data governance framework that outlines roles, responsibilities, policies, and procedures is the foundation of effective data governance. The framework should be aligned with organizational goals and supported by management. Clear communication with the organization is key.
Encouraging a data-driven culture within the organization fosters accountability and responsibility for data – how to use, access, and interpret data and knowing data are trustworthy go a long way for an end-user. Training employees on data governance policies and best practices can help promote data literacy and appropriate data usage.
Leveraging data governance tools and technologies can streamline data management processes and improve data quality. Data governance tools can automate data profiling, data quality checks, data cleansing, and data monitoring activities.
Establishing data stewardship programs ensures that data assets are managed effectively. Data stewards play a crucial role in maintaining data quality and ensuring compliance with data governance policies.
Continuously monitoring and measuring data quality is essential for maintaining high standards. Implementing data quality metrics and performance indicators can help track progress over time and identify areas for improvement.
Implementing data governance can be challenging due to various factors. Let’s take a look at what some of those factors are.
Resistance to change is a common challenge in implementing data governance. Overcoming this requires strong leadership, clear and frequent communication, and employee engagement.
Data silos can hinder effective data governance by preventing data integration and sharing. Removing data silos and promoting collaboration is essential for ensuring data access, trust, and quality.
Limited resources can challenge data governance practices. Allocating sufficient resources (time, people, finances) and prioritizing data governance initiatives can help address this challenge.
Regulatory requirements are constantly evolving, making it challenging to maintain compliance. Staying informed about regulatory changes and updating data governance practices accordingly is essential for compliance.
By addressing these challenges and implementing common practices, organizations can ensure high-quality data, foster trust in their data assets, and achieve their strategic goals.
Effective data governance is a continuous process that requires ongoing commitment and investment, but the benefits of high-quality data make it a worthwhile endeavor.
As the amount of data continues to increase, these practices must evolve to address new challenges and opportunities. Emerging trends and innovations can help shape the future of data governance, enhancing its effectiveness and making it easier to implement in your organization.
Data governance is a strategic necessity for organizations striving for data quality, compliance, and competitive advantage. Establishing robust frameworks and implementing data quality tools can help businesses make sure their data is accurate, consistent, and secure.
At SVA Consulting, we provide comprehensive data analytics, visualization, and governance solutions to help you implement best-in-class data practices. Contact us today to learn how we can assist you in your data governance journey.
© 2024 SVA ConsultingShare this post:
Jacob is the Director of Analytics with SVA Consulting. In his role, Jacob assists clients by strategically architecting solutions that help unlock meaning in data. Alongside a strong team, he designs and builds client-specific data automation and visualization solutions to fit the unique needs of a given client.
contact@svaconsulting.com
(800) 366-9091
1221 John Q Hammons Dr., Suite 201 Madison, WI 53717
18650 W. Corporate Dr., Suite 205 Brookfield, WI 53045
1600 Utica Ave S, 9th Floor
Saint Louis Park, MN 55416
7135 E. Camelback Road, 230
Scottsdale, AZ 85251
©2025 SVA Consulting, LLC. All Rights Reserved. | Privacy Policy | Cookie Policy | CCPA